Common data analysis methods
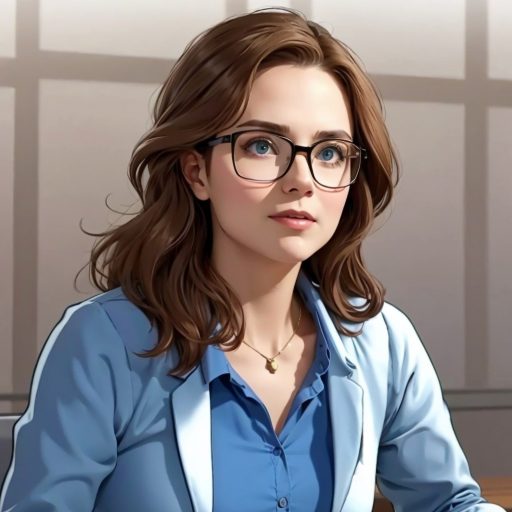
Dr Lynette Pretorius
Contact details
Dr Lynette Pretorius is an award-winning educator and researcher specialising in doctoral education, academic identity, student wellbeing, AI literacy, research skills, and research methodologies.
Unlocking meaningful insights from data starts with selecting the right analysis strategy. Each approach to data analysis offers unique pathways for understanding complex information, yet choosing the best fit depends on knowing what each method can reveal. In this post, I explore five key strategies: statistical analysis, content or document analysis, thematic analysis, phenomenological analysis, and visual analysis. By unpacking their distinct strengths and limitations, I explore how these methods empower researchers to make well-informed, impactful decisions tailored to their specific research goals. It is important to note that most qualitative data analysis methods require you to do qualitative data coding. You can read more about how to do data coding in this blog post.
Statistical Analysis
Statistical analysis is a quantitative method that processes numerical data to uncover patterns, relationships, or trends. Researchers can make inferences about populations based on data by applying statistical techniques, such as regression analysis, ANOVAs, or t-tests. Statistical analysis allows for reliable data-backed conclusions and predictions, often supporting findings with measurable probabilities or confidence intervals.
The strength of statistical analysis is in its rigour and objectivity. This method allows researchers to draw generalisable conclusions from data and provides a level of precision that is invaluable in fields like healthcare, economics, and the natural sciences. When well-designed, statistical studies yield insights that can influence policies, predict trends, and drive impactful organisational decisions. Here is an example of how statistical analysis might be conducted in a research context. Imagine a researcher wants to determine if a new medicine significantly improves patients’ recovery from illness compared to the traditional method. To do this, they select two separate groups of patients: one that receives the traditional medicine and one using the new medicine method. Both groups’ recovery times are collected over a period of time. After collecting the data, the researcher would need to apply a statistical test to compare these two groups to see if there is an observed difference that is statistically significant. A statistically different result would indicate that one medicine improved recovery time compared with the other. Statistical calculations are often done using software.
Statistical analysis has its limitations, however. One major drawback is that it requires large, high-quality datasets to yield dependable results. Small sample sizes can introduce significant errors, as they may not accurately represent the larger population, leading to misleading conclusions. Poorly collected or biased data also compromises results, as it can distort findings or exaggerate relationships that are not truly there, reducing the accuracy and generalisability of insights drawn from the analysis. Misuse or misinterpretation of statistical tools can further compound these issues. Without careful application, analysts may draw incorrect conclusions. Over-reliance on p-values or neglecting assumptions underlying statistical tests can also lead to erroneous claims. These pitfalls make statistical literacy essential for researchers, as understanding the limitations and appropriate applications of statistical techniques is crucial for producing valid and actionable results.
Content or Document Analysis
Content and document analysis is a method used to systematically review and interpret how topics, themes, and narratives are represented in texts or documents, such as news articles, speeches, policy documents, or social media posts. Researchers segment content into manageable sections, categorising them into themes to identify patterns and relationships. By systematically coding data, analysts can explore the underlying discourses, values, and beliefs present in the material, shedding light on how social issues, ideas, or viewpoints are communicated over time. Imagine a researcher is interested in understanding how the government’s language around literacy programs has evolved over the past decade, focusing on whether policies emphasise economic benefits, educational equity, or community development. The researcher would identify and collect a series of key government policy documents on literacy published over the last twenty years. A coding framework would then need to be created to categorise the main themes, keywords, and topics that appear in these documents. The researcher can then begin systematically coding each document, highlighting instances of relevant themes and noting their frequency and context. By comparing the frequency and placement of these themes, the researcher can identify patterns, such as whether the focus on literacy policy has shifted from an economic and workforce development perspective to a broader emphasis on lifelong learning and social inclusion.
One of the key strengths of content and document analysis is its ability to make sense of qualitative data in a structured, quantitative-like manner. This method allows researchers to conduct reviews efficiently, identifying trends and shifts in discourse over time or between different contexts. By examining context, document analysis reveals nuanced cultural and historical insights that might otherwise remain obscured. This approach is also highly adaptable, meaning it can be used across various fields, from sociology and media studies to political science and business research. Additionally, it enables researchers to process large volumes of textual data systematically, making it ideal for studies with extensive datasets. With a robust coding framework, content analysis also provides a replicable process, allowing other researchers to verify findings or apply similar frameworks in different contexts.
Despite its usefulness, content and document analysis have several limitations that researchers must consider. One significant drawback is the potential for researcher bias. Without a well-defined and consistently applied coding framework, results may vary considerably between different researchers or even the same researcher over time. Additionally, content analysis may fail to capture deeper, underlying meanings or nuanced insights if the categories are too rigid or simplistic, leading to an oversimplification of complex messages. Another limitation is the dependency on the quality and relevance of the chosen content; if the material does not comprehensively represent the research topic or if the data source is limited, findings may not accurately reflect broader trends or social phenomena.
Thematic Analysis
Thematic analysis is a flexible qualitative method that involves identifying, analysing, and reporting patterns or themes within data. Researchers use this approach to make sense of qualitative data by sorting segments of information into recurring themes, which can reveal insights into the attitudes, motivations, and concerns of participants. Thematic analysis is lauded for its versatility, as it can be used across a wide range of research contexts and types of qualitative data. Unlike more rigid methods, thematic analysis is adaptable and accessible, allowing researchers to approach data with a flexible coding framework. This makes it easier to derive meaningful interpretations from raw data, especially when exploring complex social or cultural issues. Furthermore, it provides an excellent balance of depth and structure, enabling researchers to gain valuable insights without requiring advanced technical skills.
There are various forms of thematic analysis, each offering unique perspectives on how themes are identified and interpreted. One well-known form that I like to use is Braun and Clarke’s reflexive thematic analysis, which emphasises the active role of the researcher in identifying, coding, and constructing themes. In this method, themes are developed through an iterative, reflective process that is deeply influenced by the researcher’s insights and engagement with the data. For example, imagine a researcher examining interviews about people’s experiences in adult literacy programs. Following this approach, the researchers would first familiarise themselves with the data, repeatedly reading transcripts to get an initial sense of participants’ responses. Next, they would begin generating initial codes, such as “empowerment,” “challenges in accessing resources,” or “improvements in confidence.” As the researchers move through the iterative stages of analysis, these codes are grouped into potential themes that are refined and reviewed against the dataset, ultimately allowing the researcher to construct meaningful, nuanced themes such as “literacy is the gateway to success”. Through this reflexive process, a rich, context-sensitive understanding that captures both the explicit and implicit meanings in participants’ narratives is developed.
On the downside, thematic analysis is susceptible to researcher bias due to the subjectivity involved in constructing themes. This subjectivity is both a strength, as it allows for rich, multifaceted interpretations, and a challenge, as it introduces inconsistencies that can complicate analysis. There is also the risk of oversimplification, as complex data may be reduced to general themes that lack the depth and detail needed to fully represent participants’ experiences or the nuances of the research topic. Thorough documentation and transparency are crucial in minimising these risks and ensuring that the analysis process is both rigorous and trustworthy. Finally, thematic analysis can be time-consuming, especially in larger datasets, as it requires careful, repeated reading and coding.
Phenomenological Analysis
Phenomenological analysis is a qualitative research method designed to uncover individuals’ lived experiences and subjective perceptions regarding a specific phenomenon. Unlike other methodologies, phenomenology prioritises personal perspectives, allowing researchers to understand the meaning and significance of phenomena as experienced by individuals themselves. Through in-depth interviews or narrative analysis, researchers gather first-hand accounts and, by reflecting on the essence of these experiences, reveal what it is like to live through particular events, conditions, or relationships.
The power of phenomenological analysis lies in its depth and authenticity, allowing researchers to explore phenomena from the insider’s perspective. By focusing on the unique context of each participant’s experience, phenomenology illuminates insights that can inform personalised interventions or support programmes tailored to the specific needs of individuals. Imagine a researcher is interested in understanding the lived experiences of casual workers in the fast food industry, particularly the challenges and feelings associated with their roles. The goal is to uncover the essence of what it is like to work in a demanding, often high-turnover environment, exploring both the daily struggles and occasional rewards that come with casual, temporary employment in fast food.
- Step 1: Transcribe and Identify Significant Statements
After gathering the data, the researcher transcribes the interviews and begins identifying significant statements or phrases that capture the core experiences of these workers. Statements like “feeling undervalued,” “pressure to work quickly under stress,” or “sense of camaraderie with coworkers” highlight key aspects of their daily lives. These meaningful phrases are then extracted from each participant’s narrative and listed as individual units of meaning. - Step 3: Develop Themes and Patterns
Next, the researcher clusters these significant statements into overarching themes that reflect shared experiences. Common themes might include “navigating stressful customer interactions,” “lack of stability and security,” and “finding support among coworkers.” Each theme represents a pattern that emerges across multiple participants, although the researcher also notes any unique perspectives that deepen the understanding of individual differences within the group. - Step 4: Describe the Essence of the Experience
Finally, the researcher synthesises these themes to describe the essence of being a casual worker in the fast food sector. This description may reveal that while workers face a lack of job security, low wages, and high levels of stress, many also experience a strong bond with their coworkers, who share similar challenges and help them get through demanding shifts. The analysis highlights the dual reality of fast food work: a job often marked by emotional and physical demands, but also by a sense of resilience and solidarity among workers who rely on each other for support.
Phenomenological analysis, while valuable for exploring personal experiences, has several limitations. One major limitation is that it typically relies on small sample sizes, as the focus is on in-depth, detailed accounts rather than broader trends. This means that while the findings may offer profound insights into individual experiences, they may not accurately represent the experiences of a larger population, limiting the ability to generalise results. Additionally, phenomenological analysis is highly dependent on participants’ abilities to articulate their feelings and experiences clearly, which can vary greatly among individuals and may lead to incomplete or skewed data. Finally, the researcher’s own biases and interpretations must be carefully managed, given the focus on individual lived experiences. This makes it essential for researchers to maintain reflexivity and transparency throughout the process.
Visual Analysis
Visual analysis is a qualitative method focused on interpreting visual data, such as photographs, paintings, videos, or digital media. Researchers use this approach to explore what images convey and how they might reflect cultural, social, or political realities. Analysts can understand how visuals communicate ideas, influence perceptions, and reinforce or challenge narratives by examining elements like composition, symbolism, and visual rhetoric.
The main strength of visual analysis is its ability to reveal insights beyond text-based data, capturing nuances that are often more immediately impactful or emotive. Visual analysis allows researchers to assess how visuals shape public opinion, influence social norms, and reflect societal values, often bringing forth perspectives that written data might overlook. Here is an example of how visual analysis on a photograph used in a social media campaign about climate change could help the researcher explore messaging about climate change.
- Step 1: Observing and Describing the Visual
Imagine the photo shows a lone polar bear stranded on a small bank of melting ice surrounded by vast, open water under a grey, clouded sky. The analyst begins by describing the scene in detail without adding interpretations. Key elements would include the polar bear’s posture, the ice floe’s size, and the cold, muted tones of the colour palette. - Step 2: Analysing the Composition and Symbolism
Next, the analyst examines the visual’s composition and symbolic meaning. The isolated bear on a shrinking piece of ice might symbolise the vulnerability of polar species in the face of climate change. The expansive ocean suggests a loss of habitat and the looming threat of extinction. The choice of muted colours and the cloudy sky could symbolise a bleak future, reinforcing the urgency of the climate message. - Step 3: Interpreting the Social and Cultural Context
Finally, the analyst interprets the broader social context. This image might aim to elicit an emotional response from viewers, compelling them to act or advocate for environmental conservation. Given the popularity of this type of imagery in climate change campaigns, the photo not only serves as a call to action but also taps into widely shared cultural understandings about climate risks and human responsibility for environmental impact. The analysis would consider how effectively this image communicates its message and resonates with the public.
However, interpreting visual data can be highly subjective, leaving significant room for varying interpretations depending on the researcher’s perspective and background. Each viewer may bring their own cultural, historical, and personal biases to the analysis, which can influence how elements within an image are perceived and understood. For accurate analysis, researchers need a strong foundation in visual literacy, including concepts like semiotics, which explores the relationship between symbols and their meanings, and symbolism, which examines how visual elements can represent abstract ideas or emotions. Understanding these concepts is essential for decoding the deeper layers of meaning within an image, yet applying them correctly can be complex and time-intensive. Additionally, the effectiveness of visual analysis is limited by the context and quality of the visuals themselves. Images captured in one context may not convey the same meanings elsewhere, as cultural interpretations can vary widely. Without sufficient contextual information, researchers may draw flawed conclusions or miss important nuances.
You can also learn more about research designs and methods by watching the videos below.
Questions to ponder
How might the researcher influence the data analysis? Is this something that should be controlled for or should this subjectivity be valued as part of the analysis process?
What challenges might arise when using visual analysis to interpret visuals from diverse cultural backgrounds, and how could researchers account for these differences in their analyses?
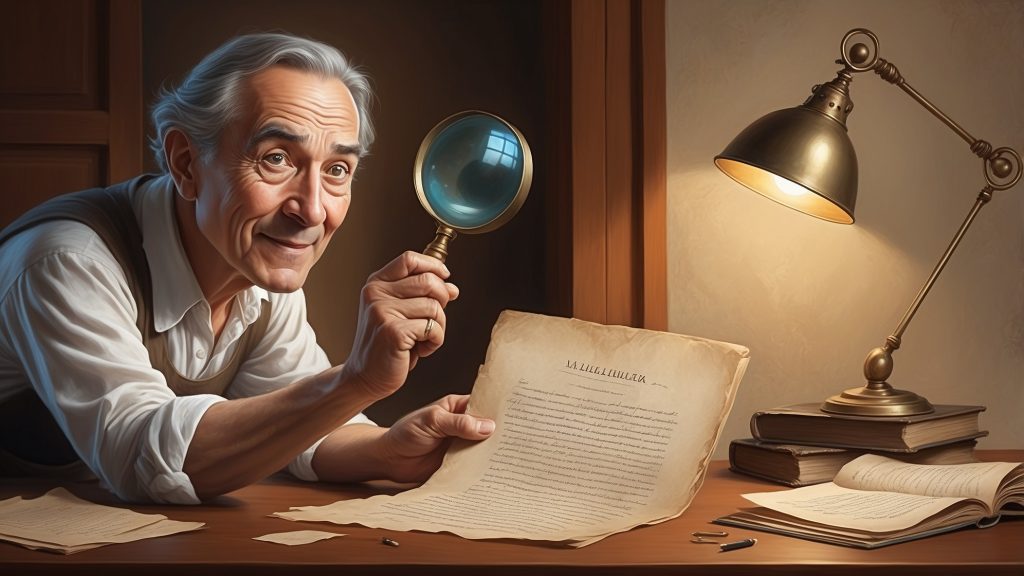
Join my 15 subscribers!
Pingback: Data coding for qualitative research – Dr Lynette Pretorius