Data coding for qualitative research
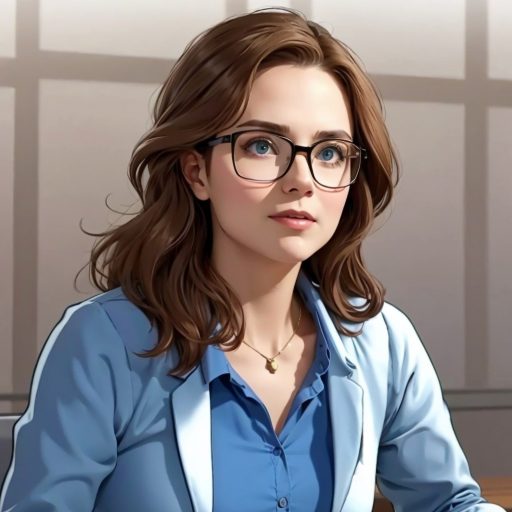
Dr Lynette Pretorius
Contact details
Dr Lynette Pretorius is an award-winning educator and researcher specialising in doctoral education, academic identity, student wellbeing, AI literacy, research skills, and research methodologies.
Coding is an essential step in transforming raw and often messy data into structured insights that reveal the nuanced layers of human experiences and perceptions. In this post, I will explore the basics of data coding. It is important to note that there is no one “correct” way to code, with different researchers preferring different approaches. As such, this post explores a general strategy that is applicable across methodologies.
What is Data Coding?
Data coding is the method by which researchers assign labels, or “codes”, to segments of data (such as lines or passages in an interview transcript). These codes categorise information and can be used to identify recurring themes, patterns, or unique insights. Unlike quantitative data, where analysis often relies on numbers, qualitative coding seeks to draw out meanings, emotions, and context. Think of coding as sorting a vast array of thoughts and words into labelled tags. Each tag represents a concept or idea that helps in making sense of the information collected. Coding provides a foundation for further analysis and interpretation, guiding researchers towards a deeper understanding of the underlying messages within their data.
Before diving into the coding process, certain preparatory steps can help clarify your objectives and streamline your approach: First, define your research questions. Knowing what you’re aiming to understand or explore will guide you towards relevant codes and themes. Second, spend time familiarising yourself with your data. Read through the data a few times to understand the overall flow and main ideas. This initial reading is crucial for getting a feel for the tone, structure, and range of content in the dataset. Third, decide on your coding approach. Decide whether you will use deductive coding (where you start with a predefined set of codes) or inductive coding (where codes emerge from the data as you go along). Inductive coding is particularly useful in exploratory studies where themes are not predetermined. Note you can use both deductive and inductive coding, which is usually the strategy I prefer. Finally, organise your work process. Whether you’re coding manually (with highlighters and notes) or digitally (with software like NVivo or MAXQDA), set up a system that allows you to easily store, retrieve, and organise your codes.
How Do You Code?
Now that you are ready to begin, here is a step-by-step approach.
Initial Coding (Open Coding):
Go through your data line-by-line or paragraph-by-paragraph and assign descriptive codes to sections that seem relevant to your research questions or themes of interest. These codes should capture the essence of each segment. The open coding stage is often exploratory, and it’s normal to have a large number of codes that may seem disconnected. Coding can feel overwhelming at this stage, especially when dealing with large volumes of data. Break down the coding process into manageable sessions and focus on specific sections.
As an example, I’ll use the coding I did for a recent paper I wrote. Let’s imagine a participant said:
“I then worked with the two co-editors to get the people who were part of the writing group to submit some abstracts for what they would like to write for a book chapter. When we received these abstracts, I was quite surprised because they actually fit quite neatly into three categories.”
As I read this quote, I can see several concepts or ideas mentioned, including collaboration, teamwork, writing groups, book authorship, chapter authorship, emotional response to texts, categorisation, and similar experiences. These can be considered inductive codes and are the ones I would assign to this sentence. This also highlights that one sentence can have multiple codes because ideas are often complex and interrelated. As I mentioned earlier, I tend to use both inductive and deductive coding approaches. To do deductive coding of these sentences, I need to use the concepts of my theoretical framework (which in this study was Communities of Practice). As such, I coded these sentences under the community element of the communities of practice framework.
Review and Refine Codes:
Once the initial coding is done, it’s time to refine. Read through your list of codes, combining those that overlap or represent similar concepts, and eliminate codes that appear redundant. This process reduces the number of codes and creates a clearer structure. For example, let’s say I had codes for teamwork, working as a team, collaborating, and working together in the overall coding of my dataset. This highlights one challenge of coding: code drift. Over time, the researcher may use slightly different wordings, or the meaning of certain codes can evolve. Keeping a codebook (a reference document that defines each code) can help maintain consistency. During this refining stage, the four codes I mentioned above can be collapsed into one code (e.g., working together) because having four separate codes for the same idea is redundant. You want to make sure that the codes you have are representative of unique concepts, even though they may be closely related.
Group Codes (Axial Coding):
Axial coding involves grouping related codes into larger categories, sometimes known as thematic clusters. At this stage, your job as the researcher is to start looking for connections between codes. Here, you’ll determine the relationships between your codes, creating categories and sub-categories that add coherence to the coded data. For example, let’s say I had codes for book authorship, chapter authorship, deciding author order, editorial decisions, and tasks in the publication process. These five ideas could be grouped into a cluster, such as “complexities of publishing“, since they are all closely related.
Further Selective Coding to Create Themes:
Once you have your categories, the final step is to create your themes. A theme represents the core idea of several of your categories, thereby giving overarching insights that help you answer your research questions. There are different approaches to creating themes, as I highlighted in another blog post, but I tend to use Braun and Clarke’s reflexive thematic analysis in my work.
Let’s look back at that initial quote I had. In the final paper, this quote was under a theme called “Same, same, but different: Everybody has a story“. This theme is most closely related to that initial code I had called similar experiences. However, while the initial code was descriptive of my data, it did not yet fully reflect the nuance and complexity of the meaning of my participants’ quotes. I had to use my deep understanding of my participants’ words to develop a theme which provided answers to my research question. When I looked back at my codes, I noticed that my participants used words like “everybody has a story” and that they noted everyone’s experiences “were all similar to each other and at the same time different from each other”. These ideas were frequently repeated, and so were clustered together during axial coding. To then create my theme, I used my participant’s words (“everybody has a story”, sometimes termed an in vivo code) and combined it with a catchy phrase (“same, same, but different”). This helped me to answer my research question, which was related to what participants learnt from reading and providing feedback on each others’ work.
It is also important to note that themes are often interrelated, reflecting the complexity of human experience. It can, therefore, be useful to create a detailed explanation for your reader of how the themes work together to address your research topic. For example, this is what I wrote in my paper to explain the connection between the first and second themes in my study:
The first theme (“same, same, but different: everybody has a story”) underscores a dual realisation among participants: while everyone brings distinct and unique life stories and perspectives to the table, there is a profound commonality in the challenges and experiences they share, particularly in the context of writing and self-reflection. The second theme (“I am not alone: everyone has problems”) is related to the first, highlighting the transformative power of shared experiences in academic settings. By recognising the commonalities in their struggles, participants felt that they were able to foster a supportive community that valued openness, mutual support, and collective growth, ultimately enhancing their PhD journey and personal development.
Final Thoughts
Coding qualitative data may seem daunting at first, but the process becomes clearer with practice. At its core, coding is about translating real human stories into research findings that can inform, inspire, and change our understanding of complex issues. Through careful, thoughtful coding, you unlock the full potential of qualitative data: capturing not just what people say, but the deeper insights hidden within their words. Happy coding!
You can also learn more about research designs and methods by watching the videos below.
Questions to ponder
What criteria might a researcher use to decide whether a code is redundant or unique enough to retain during the coding refinement phase?
What are the potential advantages and limitations of using qualitative data analysis software (like NVivo or MAXQDA) compared to manual coding?
What role does familiarity with the data (from initial readings) play in the accuracy and depth of the coding process? Could familiarity also pose any risks?
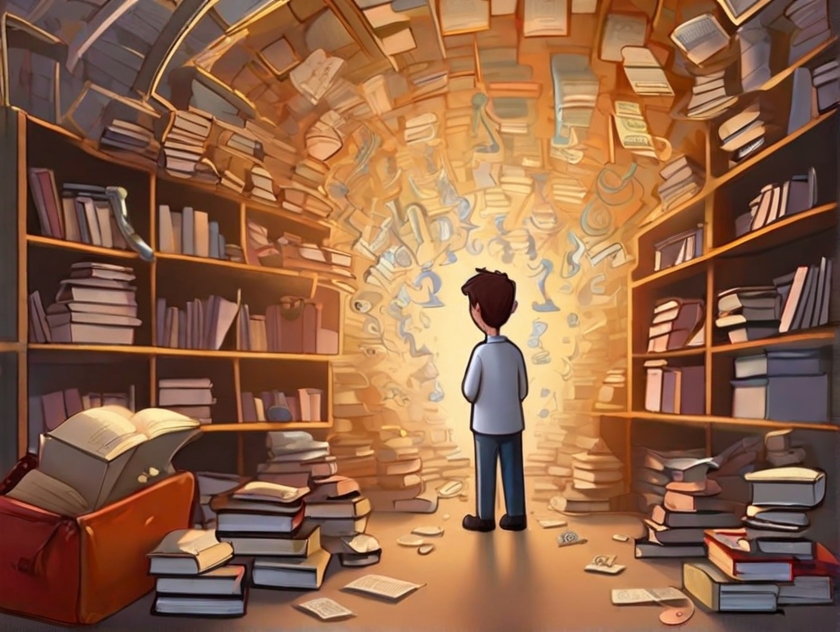
Join my 22 subscribers!
Pingback: Common data analysis methods – Dr Lynette Pretorius
Pingback: ChatGPT as a qualitative research partner – Dr Lynette Pretorius